당신은 주제를 찾고 있습니까 “r 군집 분석 – 군집분석(Clustering) – R을 활용한 계량분석 강의 노트“? 다음 카테고리의 웹사이트 https://you.tfvp.org 에서 귀하의 모든 질문에 답변해 드립니다: https://you.tfvp.org/blog/. 바로 아래에서 답을 찾을 수 있습니다. 작성자 최유진TV 이(가) 작성한 기사에는 조회수 5,832회 및 좋아요 36개 개의 좋아요가 있습니다.
r 군집 분석 주제에 대한 동영상 보기
여기에서 이 주제에 대한 비디오를 시청하십시오. 주의 깊게 살펴보고 읽고 있는 내용에 대한 피드백을 제공하세요!
d여기에서 군집분석(Clustering) – R을 활용한 계량분석 강의 노트 – r 군집 분석 주제에 대한 세부정보를 참조하세요
\”윤성사(대표: 정재훈)와 함께하는 R을 활용한 계량분석 강의 노트, 군집분석 강의\”입니다.
강의자: 최유진(강남대학교 공공인재학과 교수)
강의자 홈페이지: www.echoi0816.net
강의자 facebook: https://www.facebook.com/csueugene
r 군집 분석 주제에 대한 자세한 내용은 여기를 참조하세요.
R로 배우는 데이터분석 #22 – 군집분석 : 네이버 포스트
R로 배우는 데이터분석 #22 – 군집분석 · 군집 분석 · 은 각 개체에 대해 관측된 여러 개의 변수(x1, x2, …, xp) 값들로부터 n개의 개체를 유사한 성격을 …
Source: post.naver.com
Date Published: 4/13/2021
View: 8337
R을 사용한 K-means 군집분석 – RPubs
분석자가 설정한 K개의 군집 중심점을 랜덤하게 선정 · 관측치를 가장 가까운 군집 중심에 할당한 후 군집 중심을 새로 계산 · 기존의 중심과 새로 계산한 …
Source: rpubs.com
Date Published: 1/15/2022
View: 577
군집분석(Cluster Analysis) – AWS
몇 개의 군집으로 나누어야 하는가? 최종 결과 획득; 분석결과 시각화. 분할군집. k-means clustering; Partitioning around medos(PAM); R을 이용한 PAM.
Source: rstudio-pubs-static.s3.amazonaws.com
Date Published: 7/1/2021
View: 604
K-means clustering (R 군집분석) – BioinformaticsAndMe
K-means clustering (R 군집분석) Start. BioinformaticsAndMe. K-means clustering. : K-평균 클러스터링은 주어진 데이터를 K개의 클러스터로 묶는 …
Source: bioinformaticsandme.tistory.com
Date Published: 6/30/2021
View: 1362
[R 데이터 분석] 군집분석의 이해 :: 군집을 묶는 기준 ‘거리’ / 군집 …
군집 분석이란 모집단 또는 범주에 대한 사전 정보가 없는 경우에 주어진 관측값(레코드)들 사이의 거리 또는 유사성을 이용하여 전체를 몇개의 집단 …
Source: ybeaning.tistory.com
Date Published: 9/13/2021
View: 7619
[데이터사이언스/R] Red Wine Quality – 군집분석(Clustering …
목차> Red Wine Quality – 데이터 설명 및 분석 1. 데이터 확인 1-1. 데이터 소개 – 레드 와인의 물리 화학적 특징과 퀄리티 점수를 보여주는 CSV파일 …
Source: programmer-ririhan.tistory.com
Date Published: 2/17/2022
View: 9999
[R] 비지도 학습의 방법 : 군집분석(Clustering Analysis)
[R] 비지도 학습의 방법 : 군집분석(Clustering Analysis). kerpect 2020. 7. 21. 13:21. 320×100 … 분석유형 : 연관분석, 군집분석 → 데이터마이닝 기반.Source: kerpect.tistory.com
Date Published: 12/5/2022
View: 7815
혼합형 고객 데이터 군집분석을 통한 고객 세그먼트 결정 R 예시
연속형과 범주형을 모두 포함하고 있는 혼합형 고객 데이터 마이닝을 수행할 때에는 Gower Similarity Measure 군집분석을 사용해야 합니다. R에서 어떻게 Gower …
Source: www.theoneplanning.com
Date Published: 5/22/2021
View: 8171
주제와 관련된 이미지 r 군집 분석
주제와 관련된 더 많은 사진을 참조하십시오 군집분석(Clustering) – R을 활용한 계량분석 강의 노트. 댓글에서 더 많은 관련 이미지를 보거나 필요한 경우 더 많은 관련 기사를 볼 수 있습니다.
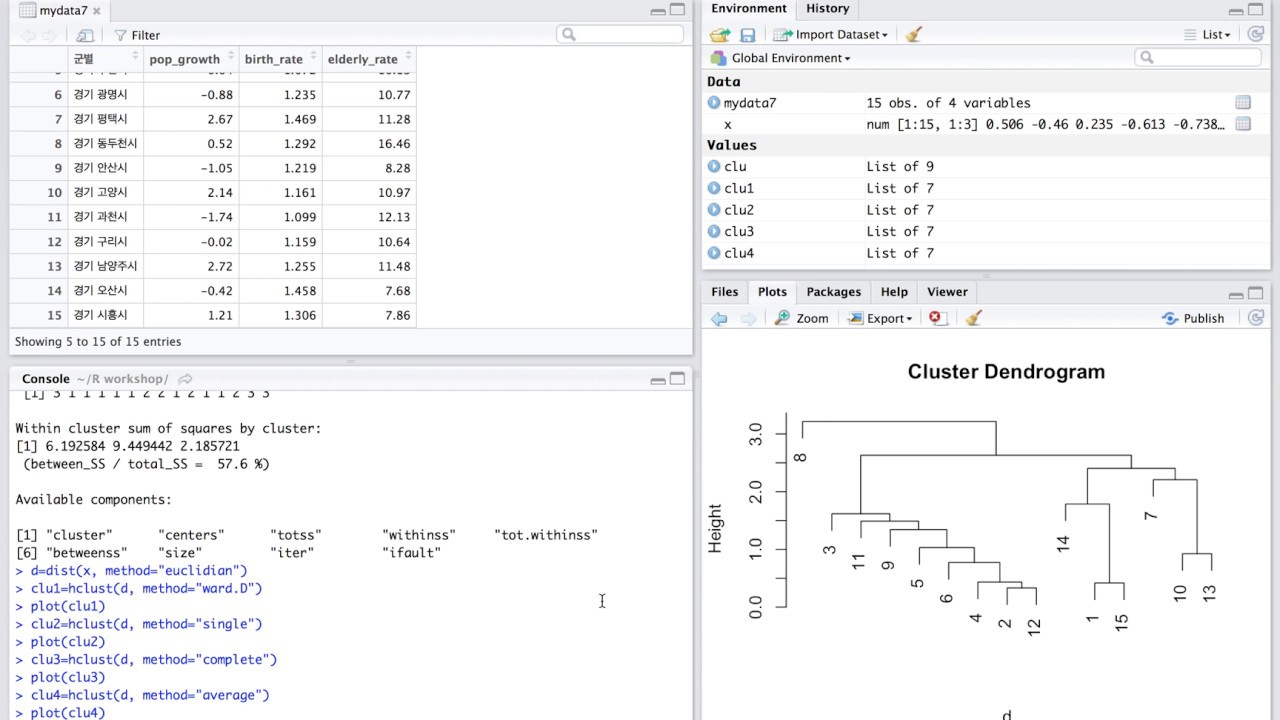
주제에 대한 기사 평가 r 군집 분석
- Author: 최유진TV
- Views: 조회수 5,832회
- Likes: 좋아요 36개
- Date Published: 2017. 3. 2.
- Video Url link: https://www.youtube.com/watch?v=RCTA8x0-lKM
K-means clustering (R 군집분석)
K-means clustering (R 군집분석) Start
BioinformaticsAndMe
K-means clustering
: K-평균 클러스터링은 주어진 데이터를 K개의 클러스터로 묶는 알고리즘
: 각 클러스터와 거리 차이의 분산을 최소화하는 방식으로 작동
: 자율 학습의 일종
: Label이 없는 입력 데이터에 Label을 표지하는 역할을 수행
K-means clustering 과정
1) 새로운 변수 설정 iris2 <- iris 2) 5번째 컬럼 제거 iris2$Species <- NULL 3) kmeans 알고리즘으로 클러스터링 3개 생성 kmeans.result <- kmeans(iris2, 3) 4) 실제 클러스터링 결과 점검을 위해, 테이블을 생성하여 비교 table(iris$Species, kmeans.result$cluster) 5) 시각화 plot(iris2[c("Sepal.Length", "Sepal.Width")], col = kmeans.result$cluster, pch=15) 6) 각 클러스터 중심 그리기 ( centers : 각 클러스터별로 컬럼의 평균값을 나타낸 것) points(kmeans.result$centers[,c("Sepal.Length", "Sepal.Width")], col= 1:3, pch=8, cex=4) #Reference 1) http://pypr.sourceforge.net/kmeans.html 2) http://ropatics.com/data-mining/r-and-data-mining/RDM-Clustering.html K-means clustering (R 군집분석) End BioinformaticsAndMe
[R 데이터 분석] 군집분석의 이해 :: 군집을 묶는 기준 ‘거리’ / 군집분석의 평가
군집 분석이란
모집단 또는 범주에 대한 사전 정보가 없는 경우에 주어진 관측값(레코드)들 사이의 거리 또는 유사성을 이용하여 전체를 몇개의 집단으로 나누는 분석이라고 할 수 있다 .
쉽게 말해 하나의 집단을 특성이 유사한 몇 개의 세부 집단으로 나누는 것이다.
[참고] https://news.joins.com/article/7085162위 사진을 보면 군집 분석에 대해 확실히 감을 잡을 수 있다.
중고등학생 시절을 되돌아 보면, 교탁앞에는 공부를 열심히하는 친구들이 주로 앉고,
뒷쪽 구석에는 공부에 관심없는 친구들이 모여 앉곤 한다. 이렇게 비슷한 성향의 사람들이 모여 앉아 그룹을 만드는데
군집 분석도 마찬가지로 주어진 개체를 비슷한 속성끼리 그룹화 시키는 것이다.
이렇게 세부적으로 그룹화 시키게 되면 , 각 집단의 성격을 파악하기 쉽고, 데이터 전체의 구조에 대한 이해를 도울 수 있다.
하지만 군집분석은 항상 옳거나 필요한 분석은 아니다. 즉, 군집분석이 의미가 없을 수 있다.
군집을 몇개로 나누어야 옳은 건가라고 했을 때, 거기에 대한 답이 정해져 있지 않다. 분석가가 선택하는 것이다.
군집분석은 비지도 학습으로 목표변수가 없다. 테스트와 트레이닝 데이터로 나눌 필요가 없다. 따라서 어떤 정답이 정해져 있지 않아 데이터 평가시에 문제가 있을 수 있다. 비교가 불가능하기 때문에 군집이 잘 나뉘었는지 평가가 어렵다는 것이다.
군집분석은 주로 고객을 세분화 할 때 쓰이는데, 몇 개의 군집들 중 특성 있는 고객을 뽑아 ‘맞춤상품’을 만들 수 있다. 각 집단별 차별화된 마케팅이 가능하다.
군집분석은 Clustering과 Segmentation 방법이 있다. Clustering 은 지금 까지 말한 군집분석 그 자체라고 할 수 있는데, 이러한 클러스터링은 몇 개로 나눌지가 참 애매하다.
하지만 Segmentation은, 몇개의 축을 기준으로 변수를 쪼개어 값들을 구분하는 방법이다. 대표적으로 RRM 모델이 있는데, Rscency(최근구매일), Frequency(구매빈도), Monetary(구매 금액)의 약자로 이 세가지 요소에 따라 각각의 고객들에게 점수를 부여한다. 고객에 대한 총점에 따라 고객들을 구분함으로써 각 그룹에 맞는 마케팅 전략을 짜는 것이다.
일반적으로 Clustering 과 Segmentation을 병행하여 분석을 시행하기도 한다.
군집분석의 특징
독립적으로 사용되지 않는다.
군집을 찾는 것 자체가 궁극적인 목적이 아님. 즉 데이터 탐색을 위해 한번 해보는 것.
각 관측치가 서로 얼마나 유사한지 측정 군집을 묶는 기준을 “거리”를 만들어 사용: Euclidean, Manhattan, Y값 기준 등등의 방법이 있고, 각 방법에 따라 결과가 아주 달라짐
거리: 군집을 묶는 기준
1. Euclidean Distance: 가장 흔히 사용되는 거리 척도로 두 관측치 사이의 직선 최단 거리를 의미. 초록색 선이 격자에 상관없이 즉, 사각형을 통과하지만 가장 빠르게 갈 수 있는 유클리디안 거리이다.
2. Menhatten Distance: 사각형 격자로 이루어진 지도에서 출발점부터 도착점 까지 건물들(사각형)을 가로지르지 않고 갈 수 있는 최단거리.
아래 그림에서 빨간선, 파란선, 노란선은 격자를 따라 거리를 통과하기 때문에 맨하탄 거리에 해당하며, 이 세 경로 모두 다 똑같은 거리이다.
[출처] http://en.wikipedia.org/wiki/Taxicab_geometry연속형일 경우
위 그림에서, 유클리디안 거리와 맨하탄 거리에서는 A와 B의 거리가 가장 가깝지만 Y값 기준 거리를 볼때는 B와 C의 거리가 가장 가깝고 A와 B의 거리가 가장 멀다.
범주형일 경우 : 두 개체가 서로 다른 범주에 속한 횟수. A = (남자, 고졸, 경기) B = (여자, 고졸, 전남) C = (남자, 대졸, 경기)
A와 B의 거리 = 2 (성별과 지역이 다름) A와 C의 거리 = 1 (학력이 다름) B와 C의 거리 = 3 (세 범주 모두 다름)
거리가 멸먼 멀수록 유사하지 않은 것이라고 할 수 있다. 즉 거리와 유사성은 상반되는 개념이다.
군집분석에서의 차원의 저주
차원이란? 기하학에서 1차원은 점, 2차원은 선, 3차원은 면이다. 데이터를 분석하는 관점에서의 차원은 각각의 개체가 가지고 있는 변수의 수를 의미한다. 예를 들어 고객 데이터에 연령, 성별, 구매금액, 거래기간, 구매카테고리수에 대한 정보가 있다면 5차원이라고 할 수 있다.
차원의 저주란,
데이터의 차원이 증가할수록 해당 공간의 크기가 기하급수적으로 증가하는 것을 의미한다. 즉, 차원이 증가할수록 데이터의 분포 분석이나 모델 추정에 필요한 샘플 데이터의 개수가 기하급수적으로 증가하는 것이다.
이런 경우에는 차원을 줄이거나 저차원으로 투영하는 것이 필요하다.
군집분석에서의 차원의 저주는 쉽게 말해 차원이 증가할수록 거리 또한 증가하는 문제를 말한다.
변수를 많이 넣을수록 가까운 개체들도 멀게 나올 가능성이 높다.
따라서 핵심 변수만 넣는 것이 좋기 때문에 변수선택을 통해 변수를 줄이도록 한다.
변수별로 단위가 다르다면?
또 다른 문제로는 변수별 단위가 다를 때는 비교가 어렵다는 것이다.
예를 들어 어떤 변수는 금액, 어떤 변수는 나이, 어떤 변수는 구매 상품 카테고리수 등등이 있다면? 변수 비교가 어려울 뿐 아니라, 단위가 큰 변수에 대해 영향력이 매우 크게 나타날 수 있다는 문제가 있을 수 있다.
이 경우에는 “표준화” 또는 “정규화” 과정을 거쳐야 한다.
표준화 (Standardization): 표준정규분포표를 구하는 식으로 변환. 표준편차를 통해 평균으로부터 얼마나 떨어져 있는 것을 의미.
정규화 (normalization): 최소값을 0으로, 최대값을 1로 만들어 0과 1사이의 값을 가지도록 변환하여 상대적으로 비교하는 방법.
일반적으로는 표준화 방법을 더 많이 사용한다.
군집분석의 평가
어떤 군집이 좋은 군집이라고 판단할 수 있을까?
군집 내 유사성이 높을 수록 (= 분산이 작다 = 거리의 합이 작다)
집단 간 차이가 클 수록 ( = 집단간 거리가 멀다)
주요 지표
Davies-Bouldin Index : 집단간 거리가 클수록, 군집내 중심과 모든 점들의 거리 평균이 클수록 좋음. DB가 낮을수록 좋음
[참고] https://ko.wikipedia.org/wiki/K-%ED%8F%89%EA%B7%A0_%EC%95%8C%EA%B3%A0%EB%A6%AC%EC%A6%98Dunn Index: 밀도가 높은 군집이 잘 나뉘어진 클러스터링 결과로 봄. D가 높을 수록 좋음.
[참고] https://ko.wikipedia.org/wiki/K-%ED%8F%89%EA%B7%A0_%EC%95%8C%EA%B3%A0%EB%A6%AC%EC%A6%98실루엣(silhouette) 기법: -1 (bad) ~ 1(good) 까지의 점수를 주는 것.
군집분석의 장단점
장점
탐색적인 기법
다양한 형태의 데이터에 적용 가능
구조가 복잡하고 변수 많은 대용량 데이터 분석에 좋음
분석 방법의 적용 용이성 분석 대상 데이터에 대해 사정정보가 거의 필요x
단점
가중치와 거리 정의에 대한 어려움
초기 군집수의 설정의 어려움: 여러번의 탐색 적인 군집분석의 절차가 필요
결과해석의 어려움: 사전에 주어진 목적이 없으므로 결과 해석이 어려울 수 있음
[참고] 실무에 써먹는 머신러닝 with R
군집분석(Clustering Analysis) : 계층적 군집 분석 (Hierarchical Clustering), K-평균 군집 분석 (K-means Clustering)
728×90
<목차>
Red Wine Quality – 데이터 설명 및 분석
1. 데이터 확인
1-1. 데이터 정제
덴드로그램으로 확인해보기위해 데이터의 수를 약 200개로 줄인다.
– 전체 데이터가 1599개 이므로 이 중 200개의 데이터만 랜덤으로 추출한다.
library(dplyr) hc<-sample_n(wine, 200) class 변수로 사용되는 quality와 rating 변수는 모델을 적용할 데이터에서는 삭제해야한다. hc_data<-subset(hc, select=-c(quality, rating)) str(hc_data) 'data.frame': 200 obs. of 11 variables: $ fixed.acidity : num 8 6.4 7 10.8 8.9 7.1 7.8 12.2 8.8 7.7 ... $ volatile.acidity : num 0.45 0.885 0.975 0.26 0.75 0.61 0.56 0.34 0.45 0.26 ... $ citric.acid : num 0.23 0 0.04 0.45 0.14 0.02 0.12 0.5 0.43 0.3 ... $ residual.sugar : num 2.2 2.3 2 3.3 2.5 2.5 2 2.4 1.4 1.7 ... $ chlorides : num 0.094 0.166 0.087 0.06 0.086 0.081 0.082 0.066 0.076 0.059 ... $ free.sulfur.dioxide : num 16 6 12 20 9 17 7 10 12 20 ... $ total.sulfur.dioxide: num 29 12 67 49 30 87 28 21 21 38 ... $ density : num 0.996 0.996 0.996 0.997 0.998 ... $ pH : num 3.21 3.56 3.35 3.13 3.34 3.48 3.37 3.12 3.21 3.29 ... $ sulphates : num 0.49 0.51 0.6 0.54 0.64 0.6 0.5 1.18 0.75 0.47 ... $ alcohol : num 10.2 10.8 9.4 9.6 10.5 9.7 9.4 9.2 10.2 10.8 ... 1-2. 거리행렬 구하기 거리 기반이므로 변수들의 값을 표준화하고 각 점들 사이의 거리(거리행렬)를 구해준다. hc_scale<-scale(hc_data) hc_dist<-dist(hc_scale) 1, 2, 3 행 데이터와 다른 데이터들과의 거리를 계산 값을 확인해볼 수 있다. as.matrix(wine_dist)[1:3,] 1 2 3 4 5 6 7 8 9 10 11 1 0.000000 2.200616 3.378307 4.058526 3.730904 6.198812 5.242537 4.058480 5.995339 4.310446 7.280152 2 2.200616 0.000000 3.492344 4.338353 2.967422 6.412352 4.515130 3.961033 5.637104 4.432912 6.891150 3 3.378307 3.492344 0.000000 2.197622 3.973361 4.107527 4.563170 1.427273 4.780674 3.114454 6.532054 12 13 14 15 16 17 18 19 20 21 22 1 5.998511 3.831569 6.089432 1.896099 7.171976 5.345357 4.818021 2.530091 5.012705 2.240985 2.572035 2 5.715539 3.929231 6.045429 3.132730 6.698603 4.833751 4.391930 3.599300 4.978711 2.047614 2.942882 3 4.639037 2.146202 6.306019 3.379558 5.694200 4.879030 4.638929 2.018307 2.194946 2.815712 2.610826 2. HC모델 적용 (계층적 군집화) hclust() 함수 : HC모델을 생성해준다. - hclust() documentation 참고 - method 옵션 을 이용하여 기준법을 설정할 수 있다. 2-1. 최단거리 기준법(single) hc_model<-hclust(hc_dist, method="single") hc_model Call: hclust(d = hc_dist, method = "single") Cluster method : single Distance : euclidean Number of objects: 200 NbClust() 함수 를 이용하여 최적의 군집 개수를 찾는다. library(NbClust) nc <- NbClust(hc_scale, distance="euclidean", min.nc=2, max.nc=15, method="single") *** : The Hubert index is a graphical method of determining the number of clusters. In the plot of Hubert index, we seek a significant knee that corresponds to a significant increase of the value of the measure i.e the significant peak in Hubert index second differences plot. *** : The D index is a graphical method of determining the number of clusters. In the plot of D index, we seek a significant knee (the significant peak in Dindex second differences plot) that corresponds to a significant increase of the value of the measure. ******************************************************************* * Among all indices: * 7 proposed 2 as the best number of clusters * 10 proposed 3 as the best number of clusters * 1 proposed 4 as the best number of clusters * 2 proposed 11 as the best number of clusters * 2 proposed 12 as the best number of clusters * 1 proposed 15 as the best number of clusters ***** Conclusion ***** * According to the majority rule, the best number of clusters is 3 ******************************************************************* → 군집 수를 3개로 설정하는 것이 10 번의 선택을 받았으므로 가장 적합하다는 결론이 나왔다. 3개의 군집으로 분류할 경우 군집별 데이터의 개수를 확인하였다. hc_cutree<-cutree(hc_model, k=3) table(hc_cutree) hc_cutree 1 2 3 198 1 1 3개의 군집으로 분류한 결과를 덴드로그램으로 시각화 하였다. plot(hc_model, hang=-1) rect.hclust(hc_model, k=3) 3개의 군집으로 분류한 결과를 산점도로 시각화 하였다. - x : volatile.acidity, y : alcohol → 상관계수가 가장 높은 상위 두 변수 사용 df<-as.data.frame(hc_scale) plot(df$volatile.acidity, df$alcohol, col=hc_cutree, pch=hc_cutree) 2-2. 최장 연결법(complete) hc_model<-hclust(hc_dist, method="complete") nc <- NbClust(hc_scale, distance="euclidean", min.nc=2, max.nc=15, method="complete") if ((resCritical[ncB - min_nc + 1, 3] >= alphaBeale) && (!foundBeale)) {에서 다음과 같은 에러가 발생했습니다: TRUE/FALSE가 필요한 곳에 값이 없습니다 추가정보: 경고메시지(들): pf(beale, pp, df2)에서: NaN이 생성되
→ 오류가 발생한다…..ㅜ
2-3. 평균 연결법(average)
hc_model<-hclust(hc_dist, method="average") nc <- NbClust(hc_scale, distance="euclidean", min.nc=2, max.nc=15, method="average") *** : The Hubert index is a graphical method of determining the number of clusters. In the plot of Hubert index, we seek a significant knee that corresponds to a significant increase of the value of the measure i.e the significant peak in Hubert index second differences plot. *** : The D index is a graphical method of determining the number of clusters. In the plot of D index, we seek a significant knee (the significant peak in Dindex second differences plot) that corresponds to a significant increase of the value of the measure. ******************************************************************* * Among all indices: * 7 proposed 2 as the best number of clusters * 5 proposed 3 as the best number of clusters * 1 proposed 7 as the best number of clusters * 1 proposed 11 as the best number of clusters * 8 proposed 12 as the best number of clusters * 1 proposed 15 as the best number of clusters ***** Conclusion ***** * According to the majority rule, the best number of clusters is 12 ******************************************************************* hc_cutree<-cutree(hc_model, k=12) table(hc_cutree) hc_cutree 1 2 3 4 5 6 7 8 9 10 11 12 135 41 2 5 2 3 1 5 1 2 1 2 plot(hc_model, hang=-1) rect.hclust(hc_model, k=12) df<-as.data.frame(hc_scale) plot(df$volatile.acidity, df$alcohol, col=hc_cutree, pch=hc_cutree) 2-4. 중심 연결법(centroid) hc_model<-hclust(hc_dist, method="centroid") nc <- NbClust(hc_scale, distance="euclidean", min.nc=2, max.nc=15, method="centroid") *** : The Hubert index is a graphical method of determining the number of clusters. In the plot of Hubert index, we seek a significant knee that corresponds to a significant increase of the value of the measure i.e the significant peak in Hubert index second differences plot. *** : The D index is a graphical method of determining the number of clusters. In the plot of D index, we seek a significant knee (the significant peak in Dindex second differences plot) that corresponds to a significant increase of the value of the measure. ******************************************************************* * Among all indices: * 7 proposed 2 as the best number of clusters * 10 proposed 3 as the best number of clusters * 2 proposed 9 as the best number of clusters * 2 proposed 11 as the best number of clusters * 2 proposed 15 as the best number of clusters ***** Conclusion ***** * According to the majority rule, the best number of clusters is 3 ******************************************************************* hc_cutree<-cutree(hc_model, k=3) table(hc_cutree) hc_cutree 1 2 3 198 1 1 plot(hc_model, hang=-1) rect.hclust(hc_model, k=3) df<-as.data.frame(hc_scale) plot(df$volatile.acidity, df$alcohol, col=hc_cutree, pch=hc_cutree) 2-5. 와드 연결법(ward.D, ward.D2) ward.D hc_model<-hclust(hc_dist, method="ward.D") nc <- NbClust(hc_scale, distance="euclidean", min.nc=2, max.nc=15, method="ward.D") *** : The Hubert index is a graphical method of determining the number of clusters. In the plot of Hubert index, we seek a significant knee that corresponds to a significant increase of the value of the measure i.e the significant peak in Hubert index second differences plot. *** : The D index is a graphical method of determining the number of clusters. In the plot of D index, we seek a significant knee (the significant peak in Dindex second differences plot) that corresponds to a significant increase of the value of the measure. ******************************************************************* * Among all indices: * 4 proposed 2 as the best number of clusters * 9 proposed 3 as the best number of clusters * 1 proposed 4 as the best number of clusters * 1 proposed 6 as the best number of clusters * 2 proposed 7 as the best number of clusters * 1 proposed 8 as the best number of clusters * 1 proposed 10 as the best number of clusters * 2 proposed 13 as the best number of clusters * 2 proposed 15 as the best number of clusters ***** Conclusion ***** * According to the majority rule, the best number of clusters is 3 ******************************************************************* hc_cutree<-cutree(hc_model, k=3) table(hc_cutree) hc_cutree 1 2 3 88 60 52 plot(hc_model, hang=-1) rect.hclust(hc_model, k=3) df<-as.data.frame(hc_scale) plot(df$volatile.acidity, df$alcohol, col=hc_cutree, pch=hc_cutree) ward.D2 hc_model<-hclust(hc_dist, method="ward.D2") nc <- NbClust(hc_scale, distance="euclidean", min.nc=2, max.nc=15, method="ward.D2") *** : The Hubert index is a graphical method of determining the number of clusters. In the plot of Hubert index, we seek a significant knee that corresponds to a significant increase of the value of the measure i.e the significant peak in Hubert index second differences plot. *** : The D index is a graphical method of determining the number of clusters. In the plot of D index, we seek a significant knee (the significant peak in Dindex second differences plot) that corresponds to a significant increase of the value of the measure. ******************************************************************* * Among all indices: * 5 proposed 2 as the best number of clusters * 3 proposed 3 as the best number of clusters * 3 proposed 4 as the best number of clusters * 1 proposed 5 as the best number of clusters * 1 proposed 6 as the best number of clusters * 5 proposed 7 as the best number of clusters * 2 proposed 11 as the best number of clusters * 2 proposed 13 as the best number of clusters * 1 proposed 15 as the best number of clusters ***** Conclusion ***** * According to the majority rule, the best number of clusters is 2 ******************************************************************* hc_cutree<-cutree(hc_model, k=2) table(hc_cutree) hc_cutree 1 2 129 71 plot(hc_model, hang=-1) rect.hclust(hc_model, k=2) df<-as.data.frame(hc_scale) plot(df$volatile.acidity, df$alcohol, col=hc_cutree, pch=hc_cutree) → 이전까지의 연결법을 사용했을 때는 모두 너무 편향적이게 분류가 되는 모습을 보였다. → 그나마 와드 연결법을 사용하였을 때 분류가 적용되는 듯한 모습을 보여준다. 3. K-means 모델 적용 library(graphics) 3-1. 군집 개수 결정 최적의 군집 개수를 확인해본 결과 3개의 군집 개수가 가장 적절 하다고 나왔다. nc <- NbClust(hc_scale, distance="euclidean", min.nc=2, max.nc=15, method="kmeans") *** : The Hubert index is a graphical method of determining the number of clusters. In the plot of Hubert index, we seek a significant knee that corresponds to a significant increase of the value of the measure i.e the significant peak in Hubert index second differences plot. *** : The D index is a graphical method of determining the number of clusters. In the plot of D index, we seek a significant knee (the significant peak in Dindex second differences plot) that corresponds to a significant increase of the value of the measure. ******************************************************************* * Among all indices: * 5 proposed 2 as the best number of clusters * 10 proposed 3 as the best number of clusters * 1 proposed 6 as the best number of clusters * 2 proposed 7 as the best number of clusters * 1 proposed 11 as the best number of clusters * 2 proposed 13 as the best number of clusters * 1 proposed 14 as the best number of clusters * 1 proposed 15 as the best number of clusters ***** Conclusion ***** * According to the majority rule, the best number of clusters is 3 3-2. K-means 모델 생성 kmeans() 함수를 이용하여 군집 개수를 3개로 나누는 k-평균 군집화 모델을 생성한다. - k-평균 군집화 또한 관측치 사이의 거리를 이용하므로 평균화한 데이터를 사용한다. km_model<-kmeans(hc_scale, 3) km_model K-means clustering with 3 clusters of sizes 36, 46, 118 Cluster means: fixed.acidity volatile.acidity citric.acid residual.sugar chlorides free.sulfur.dioxide 1 1.4327908 -0.6735567 1.1245064 0.2357906 0.7606881 -0.004900576 2 0.2631471 -0.7940938 0.7821667 0.1055560 -0.2284503 -0.183012545 3 -0.5397054 0.5150539 -0.6479822 -0.1130851 -0.1430175 0.072838965 total.sulfur.dioxide density pH sulphates alcohol 1 0.02835748 1.1946828 -1.159909668 0.8535948 -0.5849508 2 -0.24242479 -0.1020296 0.001636419 0.2129711 0.7586628 3 0.08585315 -0.3247052 0.353232820 -0.3434414 -0.1172903 Clustering vector: 1 2 3 4 5 6 7 8 9 10 11 12 13 14 15 16 17 18 19 20 21 22 23 24 25 3 3 3 1 3 3 3 1 2 2 3 3 2 3 3 3 1 3 1 3 2 3 3 3 1 26 27 28 29 30 31 32 33 34 35 36 37 38 39 40 41 42 43 44 45 46 47 48 49 50 3 3 2 2 2 2 1 1 3 2 3 3 3 3 1 2 3 2 2 2 3 3 2 1 3 51 52 53 54 55 56 57 58 59 60 61 62 63 64 65 66 67 68 69 70 71 72 73 74 75 2 2 3 3 3 3 3 1 2 1 3 3 3 2 1 3 2 2 1 3 2 3 3 3 3 76 77 78 79 80 81 82 83 84 85 86 87 88 89 90 91 92 93 94 95 96 97 98 99 100 3 2 3 3 2 3 1 3 3 1 3 1 1 3 3 2 1 2 3 3 3 3 1 3 3 101 102 103 104 105 106 107 108 109 110 111 112 113 114 115 116 117 118 119 120 121 122 123 124 125 2 3 2 3 3 3 1 3 3 2 3 3 1 1 3 3 1 2 3 1 1 3 2 1 3 126 127 128 129 130 131 132 133 134 135 136 137 138 139 140 141 142 143 144 145 146 147 148 149 150 2 3 3 2 1 1 3 3 3 2 2 3 3 3 2 2 3 3 3 3 3 3 3 3 3 151 152 153 154 155 156 157 158 159 160 161 162 163 164 165 166 167 168 169 170 171 172 173 174 175 3 2 3 3 3 2 3 3 1 3 2 1 3 2 3 1 3 3 2 1 3 3 3 3 3 176 177 178 179 180 181 182 183 184 185 186 187 188 189 190 191 192 193 194 195 196 197 198 199 200 3 3 3 3 2 3 3 3 3 2 3 3 2 2 3 3 3 1 3 1 3 1 1 2 3 Within cluster sum of squares by cluster: [1] 492.8321 285.6163 853.6879 (between_SS / total_SS = 25.4 %) Available components: [1] "cluster" "centers" "totss" "withinss" "tot.withinss" "betweenss" [7] "size" "iter" "ifault" → 3개의 군집에는 각각 36, 46, 118개의 데이터가 있다. → 각 관측치가 몇 번째 군집에 포함되어있는지 모두 나와있다. 3-3. $cluster 확인 km_model$cluster는 군집번호(Clustering vector)를 의미한다. - 각 관측치가 몇 번째 군집에 포함되어있는지를 알려준다. plot(hc_scale, col=km_model$cluster) → 세개의 군집으로 꽤 잘 분류되어 있는 모습을 확인 할 수 있다. 3-4. $totss 확인 between_SS : 군집 간 분산 정도 → 분산이 클 수록 군집끼리 확연히 분리된다. km_model$betweenss [1] 556.8638 within_SS : 군집 내 데이터의 분산 정도 → 분산이 작을 수록 군집 내의 데이터끼리 밀집해있어 군집끼리 확연히 분리된다. km_model$withinss [1] 492.8321 285.6163 853.6879 → 두번째 군집 내의 데이터들이 다른 군집의 데이터들에 비해 밀집해 있음을 알 수 있다. total_SS = between_SS + within_SS → total_SS가 클 수록 클러스터가 잘 분류되었다는 의미를 가진다. km_model$totss [1] 2189 → total_SS가 매우 작은 편인것 같다.... 3-5. 변수간 관계 확인 plot(hc_data, col=km_model$cluster) → 두 변수의 상관관계가 커질수록 군집이 명확히 분류된다. 3-6. K 변화에 따른 withinss의 변화 kmeans() 함수의 centers 옵션을 1에서 10까지 돌아가면서 적용 해본다. within <- c() for(i in 1:10){ within[i] <- sum(kmeans(hc_scale, centers = i)$withinss) } plot(1:10, within, type="b", xlab = "Number of Clusters", ylab = "Within group sum of squares") → K가 증가할수록 withinss의 값이 작아진다. → K가 증가할수록 군집 내의 분산이 작아진다. = 군집 내의 밀도가 높아진다. → 약 6개 정도에서부터 큰 감소의 차이가 심하지 않으므로 군집의 수를 6으로 결정할 수 있을 것 같다. 3-7. K 변화에 따른 betweenss의 변화 between <- c() for(i in 1:10){ between[i] <- sum(kmeans(hc_scale, centers = i)$betweenss) } plot(1:10, between, type="b", xlab = "Number of Clusters", ylab = "between group sum of squares") → K가 증가할수록 betweenss의 값이 커진다. → K가 증가할수록 군집 간의 분산이 커진다. 3-8. K 변화에 따른 정확도의 변화 정확도는 betweenss / totss * 100으로 계산 하여 K값을 1에서 10까지 변화시키면서 그 변화를 본다. → 정확도 X , 군집에 관한 평가 측도 O bet_ss <- c() for(i in 1:10){ kms <- kmeans(hc_scale , i) bet_ss[i] <- round(kms$betweenss / kms$totss * 100,1) } y_name = paste("between_ss"," ", "/", " ", "total_ss", collapse = '') par(oma=c(0,1,0,0)) # 그래프 여백 조절(하,좌,상,우) par(mgp=c(1,0.1,0)) # 그래프 내 축 여백 조절(제목, 눈금, 지표선) plot(1:10, bet_ss, type="b", xlab = "Number of Clusters", ylab = y_name, ylim=c(0,100), las = 1) → K가 증가할 수록 정확도가 높아지는 것을 확인 할 수 있다. → gap statistics 테스트 추가하기(참고) ref. - R, SAS, MS-SQL을 활용한 데이터마이닝, 이정진 - [R 분석] 계층적 군집 분석(hierarchical clustering) (tistory.com) - R을 사용한 K-means 군집분석 (rstudio-pubs-static.s3.amazonaws.com) - [R 분석] 비계층적 군집 분석(k-means clustering) (tistory.com) 728x90
[R] 비지도 학습의 방법 : 군집분석(Clustering Analysis)
728×90
반응형
비지도 학습 (Unsupervised Learning)
: 사람 없이 컴퓨터가 스스로 레이블 되어 있지 않은 데이터에 대해 학습하는 것으로 y없이 x만 이용해서 학습하는 것 입니다. 정답이 없는 문제를 푸는 것이므로 학습이 맞게 됐는지 확인할 길은 없지만, 인터넷에 있는 거의 모든 데이터가 레이블이 없는 형태로 있으므로 앞으로 기계학습이 나아갈 방향으로 설정되어 있습니다. 통계학의 군집화와 분포 추정 등의 분야와 밀접한 관련이 있습니다.
– 컴퓨터 기계학습에 의한 분석 방법
– 종속변수(y) 없음 : 입력 데이터에 정답 없음
– 분석방법 : 규칙(패턴분석) → 공학.자연과학 계열(100년)
– 분석유형 : 연관분석, 군집분석 → 데이터마이닝 기반
군집화(Clustering) – 군집분석(Clustering Analysis) : 그룹화를 통한 예측(그룹 특성 차이 분석-고객집단 이해)
: 데이터가 쭉 뿌려져 있을 때 레이블이 없다고 해도 데이터간 거리에 따라 대충 두 세개의 군집으로 이렇게 x만 가지고 군집을 학습하는 것을 군집화 라고 합니다.
군집 분석
– 종속변수(y변수)가 없는 데이터 마이닝 기법
– 유클리드 거리 기반 유사 객체 묶음
– 고객 DB -> 알고리즘 적용 -> 패턴 추출(rule) -> 근거리 모형으로 군집형성
– 계층적 군집분석(탐색적), 비계층적 군집분석(확인적)
– 주요 알고리즘 : k-means, hierarchical
군집분석 특징
– 전체적인 데이터 구조를 파악하는데 이용
– 관측대상 간 유사성을 기초로 비슷한 것 끼리 그룹화(Clustering)
– 유사성 = 유클리드 거리
유크리드 거리
: 유클리디안 거리(Euclidean distance)는 두 점 사이의 거리를 계산하는 방법으로, 이 거리를 이용하여 유클리드 공간을 정의
유클리드 거리 계산식
관측대상 p와 q의 대응하는 변량값의 차가 작으면, 두 관측대상은 유사하다고 정의하는 식
– 분석결과에 대한 가설 검정 없음(타당성 검증 방법 없음)
– 분야 : 사회과학, 자연과학, 공학 분야
– 척도 : 등간, 비율척도(연속적인 양)
군집 구성법
– 그룹간의 유사성 계산 방법
– 최단거리법, 최장거리법, 메디안법, 중심법, 그룹평균법
군집화방법
– 계층 군집화 : 가장 가까운 대상끼리 순차적으로 묶음
– 비계층 군집화 : k-평균 군집법
1. 유클리디안 거리
실습) 유클리디안 거리 계산법
① matrix 객체 생성
x <- matrix(1:9, nrow=3, byrow = T) # nrow=3 : 3행으로 만듬, byrow = T : 열 중심 -> 행 중심 x – 출력값 – [,1] [,2] [,3] [1,] 1 2 3 [2,] 4 5 6 [3,] 7 8 9
② 유클리디안 거리 생성
dist <- dist(x, method="euclidean") # method 생략가능 / euclidean : 유클리디안 거리 생성 dist - 출력값 - 1 2 2 5.196152 3 10.392305 5.196152 5.196152 : 전체 루트 (2행 - 1행 = x^2 + 2행 - 1행 = x^2 + 2행 - 1행 = x^2) 10.392305 : 전체 루트 (3행 - 1행 = x^2 + 3행 - 1행 = x^2 + 3행 - 1행 = x^2) 5.196152 : 전체 루트 (3행 - 2행 = x^2 + 3행 - 2행 = x^2 + 3행 - 2행 = x^2) ③ 유클리드 거리 계산식의 R코드 # 1행과 2행 변량의 유클리드 거리 구하기 sqrt(sum((x[1,] - x[2, ])^2)) 출력값 : 5.196152 # 1행과 3행 변량의 유클리드 거리 구하기 sqrt(sum((x[1,] - x[3, ])^2)) 출력값 : 10.3923 2. 계층적 군집분석(탐색적 분석) 실습) 유클리디안 거리를 이용한 군집화 ① 군집분석(Clustering)분석을 위한 패키지 설치 install.packages("cluster") library(cluster) ② 데이터 셋 생성 x <- matrix(1:9, nrow=3, by=T) x - 출력값 - [,1] [,2] [,3] [1,] 1 2 3 [2,] 4 5 6 [3,] 7 8 9 ③ matrix 대상 유클리드 거리 생성 함수 dist <- dist(x, method="euclidean") # method 생략가능 dist - 출력값 - 1 2 2 5.196152 3 10.392305 5.196152 ④ 유클리드 거리 matrix를 이용한 클러스터링 hc <- hclust(dist) # 클러스터링 적용 hc ⑤ 클러스터 시각화 x11() plot(hc) # 1과2 군집(클러스터) 형성 - 1 과 2 는 같은 레벨임을 보여주고, 3번은 값의 차이가 월등히 높음을 보여줍니다. - 1 과 2 유사성을 가진 가까운 것 먼저 그룹핑을 해줍니다. 실습) 신입사원 면접시험 결과 군집분석 ① 데이터 셋 가져오기 interview <- read.csv("interview.csv", header=TRUE) names(interview) - 출력값 - [1] "no" "가치관" "전문지식" "발표력" "인성" "창의력" "자격증" "종합점수" [9] "합격여부" View(interview) ② 유클리디안 거리 계산 interview_df <- interview[c(2:7)] # 2~7 열까지만 선택(가치관~자격증 점수) idist<- dist(interview_df) # no, 종합점수, 합격여부 제거 head(idist) 출력값 : [1] 3.464102 11.445523 2.449490 15.524175 3.741657 14.142136 ③ 계층적 군집분석 hc <- hclust(idist) hc 15개의 object 유클리드 거리법으로 출력 ④ 군집분석 시각화 x11() plot(hc, hang=-1) # 덴드로그램에서 음수값을 제거할 수 있음. 거리의 계산을 통해서 크기의 값을 시각화해서 보여주는데 15개를 어떻게 군집화 할 수 있을지 보여주고 있습니다. ⑤ 군집 단위 테두리 생성 rect.hclust(hc, k=3, border="red") # 3개 그룹 선정, 선 색 지정 위의 결과를 통해서 예상했던 결과가 추가적인 코드를 통해서 출력되었습니다. 실습) 군집별 특징 보기 ① 각 그룹별 서브셋 만들기 g1<- subset(interview, no==108| no==110| no==107| no==112 | no==115) g2<- subset(interview, no==102| no==101| no==104| no==106 | no==113) g3<- subset(interview, no==105| no==114| no==109| no==103 | no==111) ② 그룹 요약통계량 - 공통점 : 자격증 summary(g1) # 불합격:5, 자격증 없음 summary(g2) # 합격:5, 자격증 있음 ummary(g3) # 불합격:5, 자격증 없음+있음. table(g3$자격증) - 출력값 - 0 1 3 2 - 자격증을 가지고 있어도 불합격 하는 사람들이 존재합니다. 3. 군집 수 자르기 실습) iris 데이터 셋을 대상으로 군집 수 자르기 iris 데이터 셋 더보기 iris 데이터 셋 R에서 제공되는 기본 데이터 셋으로 3가지 꽃의 종류별로 50개씩 전체 150개의 관측치로 구성된다. iris는 붓꽃에 관한 데이터를 5개의 변수로 제공하며, 각 변수의 내용은 다음과 같다. Sepal.Length(꽃받침 길이) Sepal.Width(꽃받침 너비) Petal.Length(꽃잎 길이) Petal.Width(꽃잎 너비) Species(꽃의 종류) : 3가지 종류별 50개 (전체 150개 관측치) ① 유클리드안 거리 계산 idist<- dist(iris[1:4]) # dist(iris[, -5]) # 계층형 군집분석(클러스터링) hc <- hclust(idist) hc plot(hc, hang=-1) rect.hclust(hc, k=4, border="red") # 4개 그룹수 ② 군집 수 자르기 ghc<- cutree(hc, k=3) # stats 패키지 제공 - 3개로 그룹핑 ghc # 150개(그룹을 의미하는 숫자(1~3) 출력) ③ iris에서 ghc 컬럼 추가 iris$ghc <- ghc head(iris) - 출력값 - Sepal.Length Sepal.Width Petal.Length Petal.Width Species ghc 1 5.1 3.5 1.4 0.2 setosa 1 2 4.9 3.0 1.4 0.2 setosa 1 3 4.7 3.2 1.3 0.2 setosa 1 4 4.6 3.1 1.5 0.2 setosa 1 5 5.0 3.6 1.4 0.2 setosa 1 6 5.4 3.9 1.7 0.4 setosa 1 table(iris$ghc) # ghc 빈도수 - 출력값 - 1 2 3 50 72 28 versicolor 와 virginica 의 돤벽하게 구분이 되지 않습니다. ( 공통 되어지는 부분 존재 ) ④ 요약통계량 구하기 g1 <- subset(iris, ghc==1) summary(g1[1:4]) g2 <- subset(iris, ghc==2) summary(g2[1:4]) g3 <- subset(iris, ghc==3) summary(g3[1:4]) 4. 비계층적 군집분석 : 데이터 셋을 대상으로 계층적으로 군집분석 시각화하는 군집 수를 파악한 후 k-means 알고리즘에 군집수를 적용하여 군집별로 시각화하는 방법 실습) ggplot2 패키지에서 제공되는 diamonds 데이터 사용 diamonds 데이터 셋 더보기 diamonds 데이터 셋 ggplot2 패키지에서 제공하는 데이터 셋으로, 약 5만4천개의 다이아몬드에 관한 속성을 기록한 데이터 셋으로 53,940개의 관측치와 10개의 변수로 구성되어 있다. 주요 변수에 대한 설명은 다음과 같다. - 주요 변수 - price : 다이아몬드 가격($326 ~ $18,823) carat : 다이아몬드 무게(0.2 ~ 5.01) cut : 컷의 품질(Fair, Good, Very Good, Premium, Ideal) color : 색상(J : 가장 나쁨 ~ D : 가장 좋음) clarity : 선명도(I1 : 가장 나쁨, SI1, SI2, VS1, VS2, VVS1, VVS2, IF : 가장 좋음) x : 길이(0~10.74mm), y : 폭(0~58.9mm), z : 깊이(0~31.8mm), depth : 깊이 비율 = z / mean(x, y) ① 군집분석에 사용할 변수 추출 install.packages("ggplot2", dependencies = T) library(ggplot2) data(diamonds) dim(diamonds) 출력값 : [1] 53940 10 t <- sample(1 : nrow(diamonds),1000) # 1000개 데이터 샘플링 test <- diamonds[t, ] # 1000개 표본 추출 dim(test) 출력값 : 1000 10 head(test) # 검정 데이터 mydia <- test[c("price","carat", "depth", "table")] # 4개 칼럼만 선정 head(mydia) ② 계층적 군집분석(탐색적 분석) result <- hclust(dist(mydia), method="average") # 평균거리 이용 result plot(result, hang=-1) # hang : -1 이하 값 제거 군집 방법(Cluster method) method = "complete" : 완전결합기준(최대거리 이용) <- default(생략시) method = "single" : 단순결합기준(최소거리 이용) method = "average" : 평균결합기준(평균거리 이용) ③ 비계층적 군집분석 result2 <- kmeans(mydia, 3) # 3개 군집 수 적용. names(result2) # cluster 칼럼 확인 - 출력값 - [1] "cluster" "centers" "totss" "withinss" "tot.withinss" [6] "betweenss" "size" "iter" "ifault" result2$cluster # 각 케이스에 대한 소속 군집수(1,2,3) result2$centers # 각 군집 중앙값 - 출력값 - price carat depth table 1 5923.048 1.1164384 61.83801 57.79007 2 1579.091 0.5119504 61.60182 57.35521 3 13368.243 1.7415534 61.93010 58.17476 # 원형데이터에 군집수 추가 mydia$cluster <- result2$cluster # 1 ~ 3 중 하나의 분주에 들어가 있음 head(mydia) # cluster 칼럼 확인 ④ 변수 간의 상관계수 보기 cor(mydia[,-5], method="pearson") # 상관계수 보기 - 출력값 - price carat depth table price 1.00000000 0.91936804 0.06374813 0.09980938 carat 0.91936804 1.00000000 0.07630058 0.15321267 depth 0.06374813 0.07630058 1.00000000 -0.25949149 table 0.09980938 0.15321267 -0.25949149 1.00000000 plot(mydia[,-5]) - 상관계수 색상 시각화 install.packages('mclust') install.packages('corrgram') library(mclust) library(corrgram) # 상관성 시각화 corrgram(mydia[,-5]) # 색상 적용 - 동일 색상으로 그룹화 표시 corrgram(mydia[,-5], upper.panel=panel.conf) # 수치(상관계수) 추가(위쪽) ⑤ 비계층적 군집시각화 plot(mydia$carat, mydia$price, col=mydia$cluster) - 중심점 표시 추가 points(result2$centers[,c("carat", "price")], col=c(3,1,2), pch=8, cex=5) 728x90 반응형
혼합형 고객 데이터 군집분석을 통한 고객 세그먼트 결정 R 예시
고객 세그먼트(Customer Segment) 도출을 위한 군집분석(Clustering Analysis) 실행 시에 연속형 데이터(Numerical Data)와 범주형 데이터(Categorical Data)가 동시에 존재할 경우에는 Gower Similarity Measure 방식을 사용해야 합니다. 오늘은 샘플 데이터를 가지고 R에서 이를 실행해 보겠습니다.
R에서 gower 패키지를 사용하려면 R version 4.0.2를 설치해야 합니다. 샘플 데이터는 “Data Mining for Business Analytics” 교재에 포함되어 있는 utilities.csv 파일을 사용합니다. 동 파일은 연속형 데이터만 존재하기 때문에 임의로 “Listed” 칼럼을 추가하고, 무작위로 “Yes” 또는 “No”의 값을 주었습니다. 참고로 데이터는 22개 미국 유틸리티 회사에 관한 것입니다.
Gower 군집분석을 하기 위해서는 “gower”와 “cluster” 패키지를 설치해야 합니다. 좀 더 유저 프랜드리한 RStudio의 사용을 권고합니다.
키워드에 대한 정보 r 군집 분석
다음은 Bing에서 r 군집 분석 주제에 대한 검색 결과입니다. 필요한 경우 더 읽을 수 있습니다.
이 기사는 인터넷의 다양한 출처에서 편집되었습니다. 이 기사가 유용했기를 바랍니다. 이 기사가 유용하다고 생각되면 공유하십시오. 매우 감사합니다!
사람들이 주제에 대해 자주 검색하는 키워드 군집분석(Clustering) – R을 활용한 계량분석 강의 노트
- 최유진
- 최유진교수
- 군집분석
- 클러스터
- Clustering
- K 평균
- K means
- 계층적 군집분석
- Hierarchical Clustering
- Cluster Analysis
- R을 활용한 계량분석 강의 노트
- 계량분석
- R활용
- 통계학
- 양적연구
- 윤성사
- 정재훈
군집분석(Clustering) #- #R을 #활용한 #계량분석 #강의 #노트
YouTube에서 r 군집 분석 주제의 다른 동영상 보기
주제에 대한 기사를 시청해 주셔서 감사합니다 군집분석(Clustering) – R을 활용한 계량분석 강의 노트 | r 군집 분석, 이 기사가 유용하다고 생각되면 공유하십시오, 매우 감사합니다.